You Don’t Need AI in Your Business!
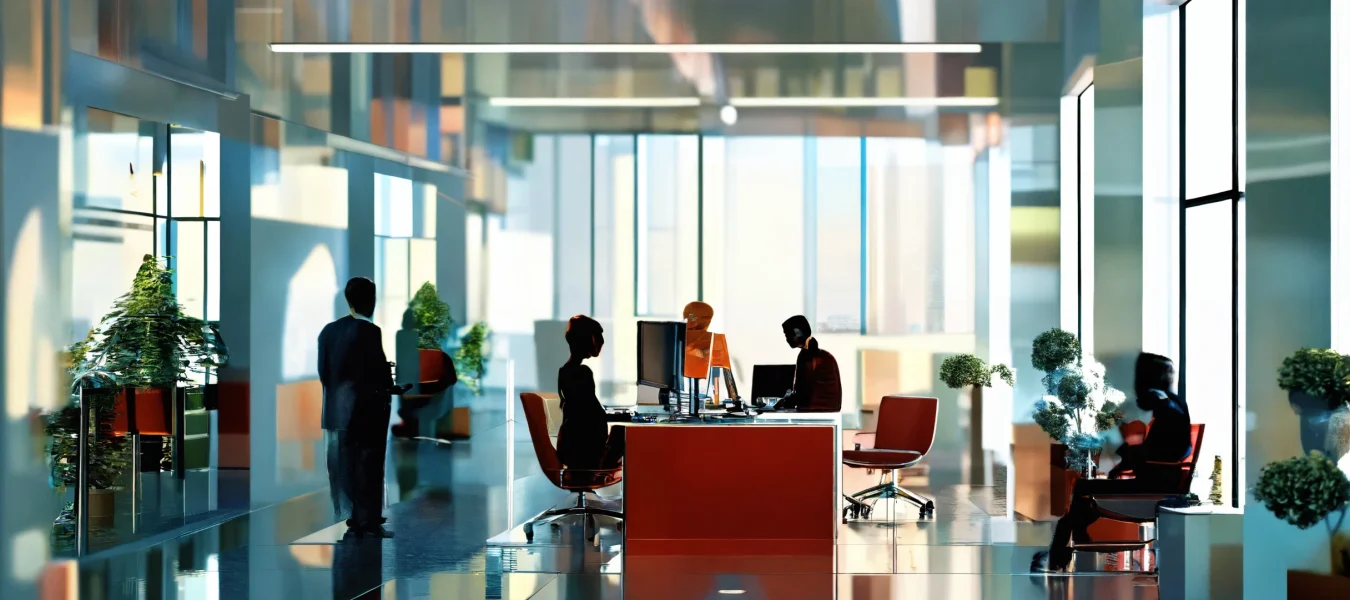
The AI Hype Train: Are You On Board or Off Track?
In the frenzied race to adopt artificial intelligence (AI), businesses are often blindly jumping on the bandwagon without critically assessing whether AI is truly necessary or beneficial for their specific needs. This headlong plunge into AI implementation can lead to costly mistakes, wasted resources, and potentially detrimental outcomes. The allure of AI’s potential is undeniable, but its indiscriminate application across all business processes is a recipe for disaster.
Consider the cautionary tale of a mid-sized European retailer that invested €2 million in an AI-driven inventory management system, only to find that it performed no better than their existing, much simpler forecasting methods. Or the U.S. healthcare provider that spent $15 million on an AI diagnostic tool that ultimately increased false positives and unnecessary treatments, leading to patient dissatisfaction and increased costs.
Before joining the AI gold rush, it’s crucial to take a step back and critically evaluate where AI can genuinely add value to your business and where it might do more harm than good. This article will explore the pitfalls of unnecessary AI adoption, the importance of process-specific implementation, and how to make informed decisions about integrating AI into your business operations.
Not Every Process Needs AI
The notion that AI can revolutionize every aspect of business operations is a dangerous misconception. In reality, many processes are better left to human expertise or simpler, more established technologies.
Consider the case of a leading Israeli fintech company that invested $3 million in an AI-powered customer service chatbot. The company hoped to reduce call center costs and improve response times. However, they soon discovered that for complex financial queries, customers strongly preferred human interaction. The AI system struggled with nuanced questions and often provided generic responses, leading to frustrated customers and a 15% drop in customer satisfaction scores.
Similarly, a German manufacturing firm implemented an AI system to optimize their production line, costing €5 million. While the system excelled at scheduling and inventory management, it faltered when dealing with sudden supply chain disruptions or unique customer requests. The rigidity of the AI system actually reduced the company’s agility, leading to missed deadlines and lost contracts.
These examples highlight a crucial point: AI is a tool, not a panacea. Before implementing AI, businesses must conduct a thorough analysis of their processes, considering factors such as:
- Complexity of decision-making required: While AI excels at data-driven decisions, it often falls short in scenarios requiring ethical judgment, contextual understanding, or interpretation of ambiguous information.
- Level of human intuition and creativity needed: Tasks involving innovation, empathy, or creative problem-solving still heavily rely on human intuition and creativity, areas where AI currently lacks sophistication.
- Potential cost savings versus implementation costs: The substantial upfront and ongoing costs of AI implementation, including data preparation and infrastructure upgrades, must be carefully weighed against potential long-term savings.
- Impact on customer satisfaction and employee morale: AI implementation can significantly affect customer experiences and employee job satisfaction, potentially leading to negative outcomes if not managed thoughtfully.
- Ability to handle exceptions and unforeseen circumstances: AI systems, trained on historical data, often struggle with unprecedented scenarios or rapidly changing environments where human adaptability remains superior.
By carefully evaluating these factors, businesses can identify areas where AI can truly add value, rather than blindly applying it across the board. The key is to view AI as a scalpel, not a sledgehammer – precision in application is crucial for success.
The Hidden Costs of Misguided AI Implementation
When businesses rush to implement AI without proper evaluation, they often underestimate the true costs involved. These expenses go far beyond the initial investment in technology and can have long-lasting impacts on the organization.
In the United States, a recent study by Gartner found that the average cost of an enterprise AI project ranges from $1.3 million to $6.7 million. However, these figures often fail to account for hidden costs. Data preparation and cleaning, for instance, can consume a significant portion of a project’s time and budget. According to a survey by Alation, data scientists spend up to 50% of their time on data preparation tasks alone.
Infrastructure upgrades present another substantial expense. Many companies need to overhaul their IT infrastructure to support AI systems, with costs potentially reaching millions of dollars. This was the case for JP Morgan Chase, which invested $12 billion in technology in 2022, with a significant portion dedicated to AI and machine learning infrastructure.
Training and upskilling employees to work with AI systems is another often-overlooked cost. Deloitte reports that 68% of executives say moderate-to-extensive training is needed to bring employees up to speed on AI systems. This training isn’t a one-time expense; as AI systems evolve, ongoing education is necessary.
Maintenance and updates for AI systems can also be surprisingly costly. Industry experts estimate that ongoing maintenance can cost up to 20% of the initial project cost annually. Google, for instance, reportedly spends hundreds of millions of dollars annually just to keep its AI models up-to-date and running efficiently.
Perhaps the most significant hidden cost is the opportunity cost. Resources devoted to unnecessary AI projects could be better spent on other strategic initiatives. This was painfully illustrated by IBM’s Watson Health division. Despite billions of dollars invested over several years, the division failed to meet its ambitious AI goals in healthcare and was eventually sold off at a significant loss.
These real-world examples underscore the importance of thorough cost-benefit analysis before embarking on AI projects. It’s crucial to consider not just the upfront costs, but also the long-term financial implications and potential risks of implementation. Sometimes, the most cost-effective solution might be to optimize existing processes or implement less complex technologies that can provide immediate, tangible benefits without the hefty price tag and risks associated with large-scale AI implementations.
The Crucial Role of Business Knowledge in AI Implementation
When integrating AI into business processes, a critical factor often overlooked is the deep, nuanced understanding of the business itself. IT professionals and AI implementers, while experts in their technical fields, may lack the intricate knowledge of business operations necessary to effectively apply AI solutions.
This knowledge gap can lead to misaligned implementations that fail to address core business needs or, worse, disrupt essential processes. A recent and pertinent example of this occurred at Zillow, the real estate marketplace, in 2021. The company implemented an AI-driven home-buying program called Zillow Offers, aimed at streamlining the process of purchasing and reselling homes.
The AI system was designed to predict home values and determine which properties Zillow should buy. However, it failed to account for the complexities and nuances of the real estate market, including regional variations, seasonal fluctuations, and the impact of the pandemic on housing trends. As a result, Zillow found itself overpaying for properties and struggling to resell them at a profit.
By November 2021, Zillow announced it was shutting down the Zillow Offers program, resulting in a $500 million write-down and laying off 25% of its workforce. This costly misstep demonstrates how even recent, sophisticated AI implementations can falter when they lack crucial business context and real-world understanding.
Bridging this gap requires a collaborative approach. Business leaders must be actively involved in the AI implementation process, not just as stakeholders, but as integral team members. They bring crucial insights into the nuances of operations, customer needs, and industry-specific challenges that might not be apparent from a purely technical perspective.
Conversely, technical teams need to develop a deeper understanding of the business contexts in which their AI solutions will operate. This might involve embedding IT professionals within business units for extended periods or creating cross-functional teams that blend technical and business expertise.
Companies like Microsoft have recognized this need and have started to create roles like “AI Business School” that bridge the gap between technical capabilities and business needs. These initiatives ensure that AI implementations are not just technically sound, but also aligned with strategic business objectives and real-world market conditions.
The lesson is clear: successful AI implementation isn’t just about having the most advanced technology. It’s about understanding how that technology can be applied in ways that genuinely enhance business operations and add value, particularly in the rapidly evolving landscape of 2023-2024. Without this crucial business knowledge, even the most sophisticated AI systems risk becoming expensive, underutilized tools rather than transformative business assets.
Understanding the Limitations of AI: Not a Silver Bullet
As we navigate the AI landscape in 2024, it’s crucial to recognize that despite its rapid advancements, AI still has significant limitations. These constraints are often overlooked in the rush to implement AI solutions, leading to unrealistic expectations and potential disappointments.
One of the most prominent limitations is AI’s struggle with context and nuance, particularly in complex decision-making scenarios. For instance, in 2023, Morgan Stanley faced regulatory scrutiny when its AI-powered wealth management system failed to adequately consider clients’ risk tolerances and financial situations. The system, while efficient at processing vast amounts of financial data, lacked the nuanced understanding of individual client needs that human advisors possess. This led to inappropriate investment recommendations for some clients, highlighting the continued importance of human oversight in sensitive financial matters.
Another limitation is AI’s dependence on historical data, which can perpetuate existing biases or become quickly outdated in rapidly changing environments. The COVID-19 pandemic provided a stark example of this. Many AI-driven supply chain management systems, trained on pre-pandemic data, failed to adapt to the sudden shifts in consumer behavior and global logistics disruptions. Companies like Walmart and Amazon had to quickly revert to more manual, human-driven decision-making processes to navigate the unprecedented market conditions.
Ethical considerations also pose significant challenges. In 2023, several major tech companies, including Google and Microsoft, faced backlash over their AI language models generating biased or inappropriate content. This underscores the ongoing struggle to create AI systems that can navigate the complex ethical landscape of human communication and decision-making.
Moreover, AI systems often lack transparency in their decision-making processes, creating a “black box” problem. This became evident in healthcare, where AI diagnostic tools, despite showing promise, faced adoption challenges due to the inability to explain their reasoning. Doctors and patients alike were hesitant to trust diagnoses without understanding the underlying logic, leading to slower-than-expected adoption rates in clinical settings.
Energy consumption is another often-overlooked limitation. Training and running large AI models require substantial computing power, leading to significant energy costs and environmental impacts. A 2023 study by the University of Massachusetts Amherst found that training a single large AI model can emit as much carbon as five cars over their lifetimes. This has led to growing concerns about the sustainability of widespread AI adoption, particularly for smaller businesses with limited resources.
Recognizing these limitations is not about dismissing AI’s potential, but rather about setting realistic expectations and identifying where AI can truly add value. In many cases, a hybrid approach that combines AI capabilities with human expertise yields the best results. For example, in legal tech, companies like LexisNexis have found success by using AI to assist with initial document review and research, while leaving complex legal reasoning and strategy to human lawyers.
As we move forward, it’s essential to approach AI implementation with a clear understanding of both its capabilities and its limitations. This balanced perspective will help businesses make informed decisions about where and how to deploy AI effectively, rather than viewing it as a one-size-fits-all solution to every business challenge.
Identifying the Right Processes for AI Implementation
While AI isn’t suitable for every business process, there are certainly areas where it can provide significant value. The key is to identify these processes carefully and implement AI solutions judiciously. Let’s explore some of the areas where AI has proven particularly effective in recent years.
Data Classification and Analysis: AI excels at processing and categorizing vast amounts of data quickly. For instance, JPMorgan Chase implemented an AI system called COiN (Contract Intelligence) in 2023, which can review commercial loan agreements in seconds, a task that previously took 360,000 hours of lawyer time annually. This application of AI not only saved time but also improved accuracy and consistency in document review.
Repetitive Tasks: AI can significantly enhance efficiency in repetitive, rule-based tasks. In 2023, UiPath, a leading robotic process automation company, helped a major telecommunications provider automate its customer onboarding process. The AI-driven system reduced processing time by 85% and errors by 95%, demonstrating the power of AI in handling high-volume, routine tasks.
Basic Decision-Making: For operational decisions that follow clear rules, AI can be highly effective. Amazon’s AI-powered inventory management system, continuously refined since its initial implementation, now predicts demand patterns and optimizes stock levels across its vast network of warehouses. In 2023, this system helped Amazon reduce its inventory costs by $10 billion while maintaining high availability of products.
Customer Service: AI-powered chatbots and virtual assistants have come a long way in recent years. In 2023, Vodafone’s AI chatbot, TOBi, handled over 70% of customer inquiries across 13 countries, significantly reducing wait times and improving customer satisfaction scores.
Predictive Maintenance: In manufacturing and infrastructure, AI has proven valuable in predicting equipment failures. In 2023, Siemens reported that its AI-driven predictive maintenance system reduced unplanned downtime by up to 30% in power plants and industrial facilities worldwide.
However, it’s crucial to note that even in these areas, AI is often most effective when used as a tool to augment human capabilities rather than replace them entirely. The human element remains vital for handling exceptions, making nuanced decisions, and providing the empathy and creativity that AI still lacks.
Moreover, the decision to implement AI should always be based on a thorough cost-benefit analysis and a clear understanding of the specific business needs and challenges. What works for one company may not be suitable for another, even within the same industry.
As we navigate the AI landscape in 2024 and beyond, the most successful organizations will be those that can strategically identify where AI can add the most value, while recognizing where human skills and judgment remain indispensable.
One significant limitation of AI is its dependency on data. AI systems require vast amounts of high-quality, relevant data to function effectively. If the data is biased, incomplete, or inaccurate, the AI’s outputs will be similarly flawed. For example, a study highlighted by McKinsey shows that biased data in AI-driven recruitment tools can lead to discriminatory hiring practices, where the system inadvertently favors certain demographic groups over others.
Another limitation is the lack of contextual understanding. AI systems can process and analyze data at scale but often struggle with tasks that require nuanced understanding or complex judgment. For example, while AI can analyze customer feedback for sentiment, it may not fully grasp cultural subtleties or idiomatic expressions that a human would easily understand. This limitation can result in misinterpretations that impact customer relations and decision-making processes.
Moreover, AI systems lack the creativity and emotional intelligence that humans naturally possess. Tasks requiring empathy, innovation, and the ability to navigate complex social interactions are currently beyond the reach of AI. For instance, while AI can assist in diagnosing medical conditions by analyzing patterns in data, the compassionate communication of a diagnosis and treatment plan still relies on the human touch.
Finally, AI systems can be vulnerable to adversarial attacks, where malicious inputs can manipulate the system into making incorrect decisions. This vulnerability poses significant risks in critical applications, such as autonomous vehicles or financial trading systems, where incorrect decisions can have severe consequences.
Understanding these limitations is crucial for businesses considering AI adoption. It helps set realistic expectations and guides companies in selecting appropriate processes for AI integration. By recognizing where AI excels and where it struggles, businesses can avoid over-reliance on technology and ensure that AI complements rather than compromises their operations.
AI as an Assistive Tool: Augmenting Human Capabilities
While the hype around AI often focuses on its potential to replace human workers, the most successful implementations in 2024 are those that use AI to augment and enhance human capabilities rather than replace them entirely.
Take the healthcare sector, for instance. In 2023, Mayo Clinic expanded its use of AI-assisted diagnostic tools. These tools don’t make diagnoses independently but instead provide radiologists with a “second opinion,” flagging potential issues in medical images that might be overlooked. This collaborative approach between AI and human experts has led to a 20% increase in early detection rates for certain cancers while reducing the workload on overextended medical staff.
In the financial sector, Goldman Sachs has been refining its AI-assisted trading platform since its initial rollout. As of 2024, the system doesn’t make trades autonomously but instead provides traders with real-time market insights and predictive analytics. This has enabled human traders to make more informed decisions, leading to a 15% improvement in trade performance compared to traditional methods.
Even in creative fields, AI is proving to be a valuable assistant. Adobe’s AI-powered tools in Creative Cloud, continually updated throughout 2023, now offer features like intelligent photo editing and AI-generated design suggestions. However, these tools don’t replace designers; instead, they handle time-consuming tasks like image tagging and basic retouching, freeing up creatives to focus on higher-level conceptual work.
The key to success in these cases is the careful integration of AI capabilities with human expertise. AI excels at processing vast amounts of data, identifying patterns, and performing repetitive tasks with consistency. Humans, on the other hand, bring creativity, emotional intelligence, and the ability to make nuanced judgments in complex situations.
By leveraging AI as an assistive tool, businesses can enhance productivity, improve decision-making, and free up human workers to focus on higher-value tasks that require uniquely human skills. This approach not only maximizes the benefits of AI but also addresses many of the concerns about job displacement.
However, implementing AI as an assistive tool requires careful planning and ongoing adjustment. It’s crucial to provide adequate training to employees on how to work effectively with AI systems. Moreover, businesses need to be prepared to continually refine and update their AI tools as technology evolves and business needs change.
As we move forward, the most successful organizations will likely be those that can strike the right balance between AI capabilities and human skills, creating a synergy that enhances overall performance and innovation.
Conclusion: A Balanced Approach to AI Implementation
As we navigate the complex landscape of AI in 2024, it’s clear that a nuanced, balanced approach to implementation is crucial for business success. The key takeaway is not that businesses should avoid AI altogether, but rather that they should approach its adoption with careful consideration and strategic planning.
Firstly, it’s essential to recognize that not every process in your business needs AI. The most successful organizations are those that have conducted thorough assessments to identify where AI can truly add value. This might be in data analysis, customer service, predictive maintenance, or other areas where AI’s strengths in processing large amounts of data and identifying patterns can be leveraged effectively.
Secondly, the hidden costs of AI implementation cannot be overlooked. From data preparation and infrastructure upgrades to ongoing maintenance and employee training, the full cost of AI often extends far beyond the initial investment. Companies must conduct comprehensive cost-benefit analyses that account for these hidden expenses to ensure that AI implementations deliver real value.
Thirdly, the importance of deep business knowledge in AI implementation cannot be overstated. The Zillow case study serves as a stark reminder that even technologically advanced AI systems can fail when they lack crucial business context. Successful AI implementation requires a collaborative approach that brings together technical expertise and in-depth understanding of business operations and market dynamics.
Furthermore, it’s crucial to understand and account for the current limitations of AI. While AI has made significant strides, it still struggles with context, nuance, and ethical considerations. Recognizing these limitations allows businesses to implement AI in ways that complement human skills rather than attempting to replace them entirely.
Finally, the most effective AI implementations are often those that use AI as an assistive tool to augment human capabilities. By leveraging AI to handle data-intensive and repetitive tasks, businesses can free up their human workforce to focus on areas that require creativity, emotional intelligence, and complex decision-making.
As we look to the future, it’s clear that AI will continue to play an increasingly important role in business. However, the path to successful AI adoption is not through blind implementation, but through thoughtful, strategic integration that aligns with business goals and complements human skills.
The businesses that will thrive in this new era will be those that can critically evaluate where AI can add value, implement it effectively, and continuously adapt their approach as technology and business needs evolve. Remember, the goal is not to use AI everywhere, but to use it wisely where it can truly enhance your business operations and outcomes.
In conclusion, while you may not need AI in every aspect of your business, understanding where and how to leverage it effectively could be the key to maintaining a competitive edge in our rapidly evolving digital landscape.